Learning over Graphs
Abstract
This talk explains how agents can learn from dispersed information and solve inference tasks of varying degrees of complexity through localized processing. The presentation also shows how information/misinformation is diffused over graphs, how beliefs are formed, and how the graph topology helps resist/enable manipulation. Examples will be considered in the context of social learning, teamwork, distributed optimization, and adversarial behavior.
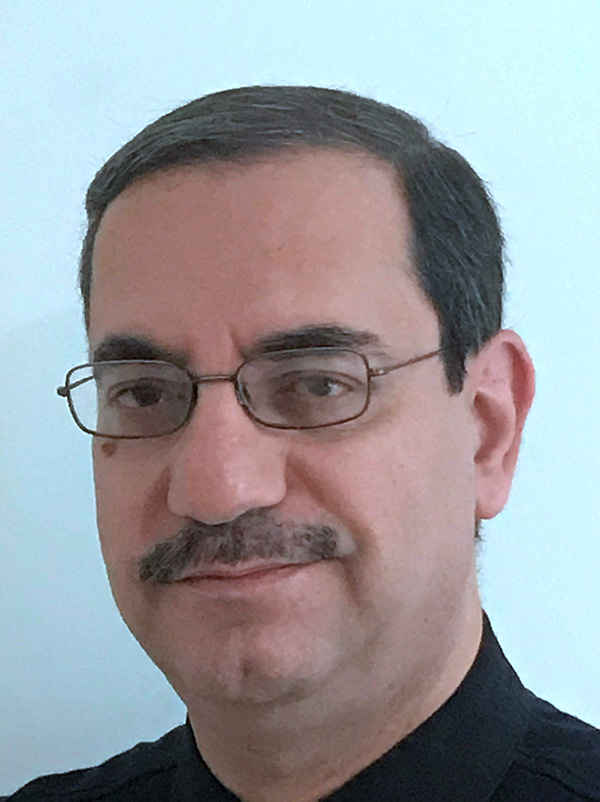
A. H. Sayed is Dean of Engineering at EPFL, Switzerland. He has served as distinguished professor and chairman of electrical engineering at UCLA where he founded the Adaptive Systems Laboratory in 1996. He is recognized as a Highly Cited Researcher and is a member of the US National Academy of Engineering. He served as President of the IEEE Signal Processing Society in 2018 and 2019. An author of over 570 scholarly publications and six books, his research involves several areas including adaptation and learning theories, data and network sciences, statistical inference, information processing theories, and multi-agent systems. His work has been recognized with several major awards including the 2020 Norbert Wiener Society Award and the 2015 Education Award from the IEEE Signal Processing Society, the 2014 Papoulis Award from the European Association for Signal Processing, the 2013 Meritorious Service Award, and the 2012 Technical Achievement Award from the IEEE Signal Processing Society, the 2005 Terman Award from the American Society for Engineering Education, the 2005 Distinguished Lecturer from the IEEE Signal Processing Society, the 2003 Kuwait Prize, and the 1996 IEEE Donald G. Fink Prize. He has been awarded several Best Paper Awards from the IEEE (2002, 2005, 2012, 2014) and EURASIP (2015) and is a Fellow of IEEE, EURASIP, and the American Association for the Advancement of Science (AAAS).