Statistical to Causal AI
Abstract
In machine learning, we use data to automatically find dependences in the world, with the goal of predicting future observations. Most machine learning methods build on statistics, but one can also try to go beyond this, assaying causal structures underlying statistical dependences. Can causal knowledge help us produce intelligent behavior? We argue that causality can play a central role in addressing some of the hard open problems of machine learning, due to the fact that causal models are more robust to changes that occur in real world datasets. We discuss implications of causal models for machine learning tasks, focusing on an assumption of ‘independent mechanisms’, and discuss an application in the field of exoplanet discovery.
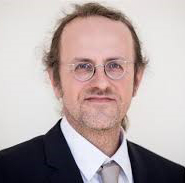
Bernhard Schölkopf's scientific interests are in machine learning and causal inference. He has applied his methods to a number of different fields, ranging from biomedical problems to computational photography and astronomy. Bernhard has researched at AT&T Bell Labs, at GMD FIRST, Berlin, and at Microsoft Research Cambridge, UK, becoming a Max Planck director in 2001. He has (co-)received the J. K. Aggarwal Prize, the Academy Prize of the Berlin-Brandenburg Academy of Sciences and Humanities, the Royal Society Milner Award, the Leibniz Award, the Koerber European Science Prize, and the BBVA Foundation Frontiers of Knowledge Award. He is Fellow of the ACM and of the CIFAR Program "Learning in Machines and Brains", a member of the German Academy of Sciences, and a Professor at ETH Zurich.
Bernhard co-founded the series of Machine Learning Summer Schools, the ELLIS grassroot initiative, and is co-editor-in-chief for the Journal of Machine Learning Research, an early development in open access and today the field's flagship journal.